The Rise of AI in Cloud Security: Game-Changing Tools and Techniques for 2024
As we dive deeper into the digital age, the intersection of artificial intelligence (AI) and cloud security is becoming a hotbed of innovation. In 2024, we’re seeing AI revolutionize how we protect our cloud environments, offering unprecedented levels of threat detection and prevention. Let’s explore the game-changing tools and techniques that are reshaping the landscape of cloud security.
How AI and Machine Learning are Revolutionizing Threat Detection in Cloud Environments
Gone are the days when security teams could rely solely on rule-based systems to detect threats. The sheer volume and sophistication of today’s cyber attacks demand a more intelligent approach. Enter AI and machine learning (ML).
These technologies are transforming threat detection in several key ways:
- Pattern Recognition: AI algorithms can analyze vast amounts of data to identify patterns that might indicate a security threat. Unlike traditional systems, AI can detect subtle anomalies that human analysts might miss.
- Behavioral Analysis: ML models can learn what constitutes “normal” behavior in your cloud environment. Any deviation from this norm can be flagged for investigation, allowing for the detection of novel threats.
- Predictive Analysis: By analyzing historical data and current trends, AI can predict potential future attacks, allowing for proactive rather than reactive security measures.
- Real-time Threat Intelligence: AI systems can continuously update their knowledge base with the latest threat intelligence, ensuring that your cloud security is always prepared for emerging threats.
Real-world Examples of AI-powered Security Tools in Action
Let’s look at some concrete examples of how AI is being used in cloud security:
- Microsoft Azure Security Center: This tool uses ML to detect and prevent threats across hybrid cloud workloads. It analyzes millions of signals to identify and prioritize potential security incidents.
- Amazon GuardDuty: AWS’s threat detection service uses ML algorithms to identify unexpected and potentially unauthorized and malicious activity within your AWS environment.
- Google Cloud’s Security AI Workbench: This platform leverages Google’s AI and ML capabilities to help security teams investigate threats, hunt for vulnerabilities, and automate response actions.
- IBM QRadar Advisor with Watson: This AI-powered tool helps security analysts investigate and respond to threats faster by automating the threat intelligence gathering process.
The Potential Future of AI in Predicting and Preventing Cloud Security Breaches
As AI continues to evolve, its role in cloud security is set to become even more significant. Here are some potential future developments:
- Autonomous Security Systems: AI could potentially manage entire security operations autonomously, from threat detection to response and mitigation.
- Advanced Threat Prediction: Future AI systems might be able to predict cyber attacks with a high degree of accuracy, perhaps even identifying potential attackers before they strike.
- AI-powered Deception Technology: AI could manage sophisticated honeypots and other deception techniques, luring attackers into revealing their methods.
- Quantum-resistant Cryptography: As quantum computing threatens to break current encryption methods, AI could play a crucial role in developing and managing quantum-resistant cryptographic systems.
Challenges and Considerations When Implementing AI-based Security Solutions
While the potential of AI in cloud security is enormous, it’s not without its challenges:
- Data Privacy Concerns: AI systems require vast amounts of data to function effectively. This raises questions about data privacy and compliance with regulations like GDPR.
- The AI Skills Gap: There’s a significant shortage of professionals who understand both AI and cybersecurity. Organizations need to invest in training and recruitment to bridge this gap.
- False Positives: AI systems, especially in their early stages, may generate false positives. Fine-tuning these systems to reduce false alarms while maintaining sensitivity to real threats is an ongoing challenge.
- The Arms Race with Cybercriminals: As defenders use more sophisticated AI, attackers are also leveraging AI to create more advanced threats. It’s a constant race to stay ahead.
- Explainability and Trust: Many AI systems, particularly deep learning models, operate as “black boxes.” Building trust in these systems and making their decisions explainable to humans is crucial for widespread adoption.
As we look to the future, it’s clear that AI will play an increasingly central role in cloud security. By embracing these technologies thoughtfully and addressing the associated challenges, organizations can significantly enhance their security posture in the cloud.
Relevant Links
- Introduction to Azure Security Center
- Amazon GuardDuty Features
- Google Cloud Security AI Workbench
- IBM QRadar Advisor with Watson
- NIST Guidelines on AI in Cybersecurity
Azure Machine Learning Use Case Diagram Guidance
To illustrate the concepts discussed in this article, consider creating a diagram that shows the flow of data and decision-making in an AI-powered cloud security system using Azure Machine Learning. Here’s a sample use case to guide your diagram:
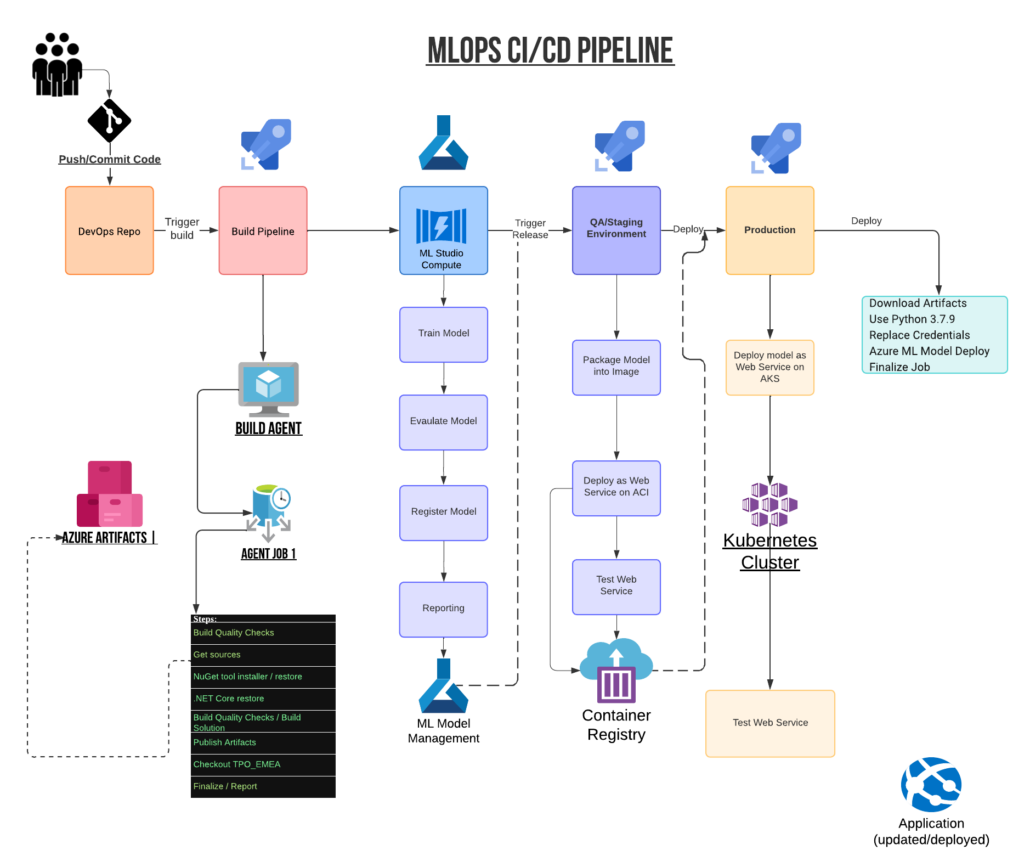
For all your storage and upgrade needs, OWC/MacSales offers reliable and high-performance solutions.
Check them out here and boost your system’s efficiency.
Leave a Reply
Want to join the discussion?Feel free to contribute!